- * 프린트는 Chrome에 최적화 되어있습니다. print
인공지능대학원 / Graduate School of Artificial Intelligence
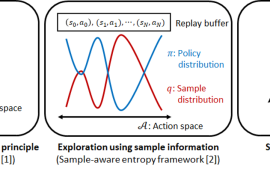
The research of our group focuses on the development of breakthrough machine learning (ML) algorithms, theoretical improvements based on mathematics, and real-world ML applications for industrial automation. In order to conduct influential research in the core areas of artificial intelligence (AI), we mainly consider the following research topics:
- Reinforcement learning
- Transfer learning/Domain adaptation
- Imitation learning
- Multi-agent systems
- Statistical learning
- Intelligent control systems
- Signal processing
Major research field
Reinforcement learning, Transfer learning/Domain adaptation, Imitation learning, Multi-agent systems, Statistical learning
Desired field of research
Real-world AI application, General machine learning, Intelligent control system