- * 프린트는 Chrome에 최적화 되어있습니다. print
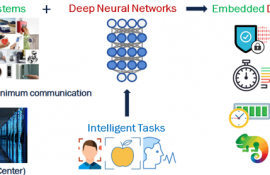
Embedded Artificial Intelligence (EAI) Lab aims to make resource-constrained real-time and embedded sensing systems capable of learning, adapting, and evolving, which facilitates Embedded AI. We have a vision that Embedded AI will enable billions of computing devices to learn, evolve, and adapt by themselves without requiring any help from other systems or humans. When those self-learning devices are connected together, they will be able to facilitate collective intelligence by learning from each other and exchanging the knowledge learned on each device, providing better insights and making better decisions. Our lab pursues excellence in research by relentlessly pushing forward state-of-the-art science and technology via disruptive ideas, game-changing perspectives, and collaboration. In our lab, we find exciting research problems and solve them, making the world a better place with real impacts. We believe in the future and people and enjoy our research. We try to be the first to take the challenge, overcome the difficulties, solve the problems, and make the world a better place.
Major research field
Embedded AI, Machine Learning, Mobile Computing, AIoT (AI + IoT), Cyber-Physical Systems
Desired field of research
Embedded AI, On-Device Machine Learning, Mobile Computing, Smart Sensing Systems, Collaborative and Federated Learning
Research Keywords and Topics
Embedded AI
Machine Learning
Mobile Computing
Real-time Systems
Smart Sensing Systems
AIoT (AI + IoT)
Cyber-Physical Systems
Research Publications
[IPSN 2023] Seulki Lee, "MicroDeblur: Image Motion Deblurring on Microcontroller-based Vision Systems", In the 22th International Conference on Information Processing in Sensor Networks, 2023.
[MobiSys 2020] Seulki Lee and Shahriar Nirjon, "Fast and Scalable In-memory Deep Multitask Learning via Neural Weight Virtualization", In the 18th ACM International Conference on Mobile Systems, Applications, and Services, 2020.
[RTAS 2020] Seulki Lee and Shahriar Nirjon, "SubFlow: A Dynamic Induced-Subgraph Strategy Toward Real-Time DNN Inference and Training", In the 26th IEEE Real-Time and Embedded Technology and Applications Symposium, 2020.
[SenSys 2019] Seulki Lee and Shahriar Nirjon, “Neuro.ZERO: A Zero-Energy Neural Network Accelerator for Embedded Sensing and Inference Systems”, In the 17th ACM Conference on Embedded Networked Sensor Systems, 2019.
Patents
Method for measuring angles between displays and electronic device using the same six-axis sensors”, Korea, Patent No.10-2015-0129196, Seulki Lee, 2015-09
국가과학기술표준분류
- EE. 정보/통신
- EE02. 소프트웨어
- EE0201. 임베디드 S/W
국가기술지도분류
- 정보-지식-지능화 사회 구현
- 011300. 차세대 정보시스템기술
녹색기술분류
- 고효율화기술
- 전력효율성 향상
- 323. 그린 IT기술
6T분류
- IT 분야
- 정보처리 시스템 및 S/W
- 010316. 기타 정보처리시스템 및 S/W 기술